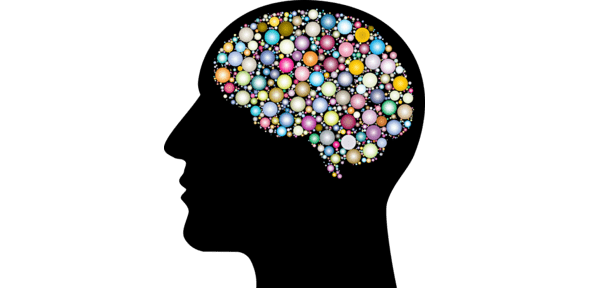
The CMIH Hub Partnership Fund has been used to support pump-priming/scoping projects with new collaborations and funding. In the second of a series of articles about these projects Dr. Michael Roberts and Dr Harpreet Hyare explain how an award from the Partnership Fund aided their research.
Applying machine learning to brain tumours for paediatric and young adult patients
What made you decide to apply to the CMIH Partnership Fund?
This project was a passion project for Harpreet, myself and too many other researchers to name, who helped kick it off in the middle of 2020. Our belief was that as machine learning (ML) and AI are leading to lots of research in adults, teenage and young adult (TYA) brain tumour patients are under-served currently.
We spent 3 years obtaining ethical approval, curating data and ensuring it could be processed in Cambridge securely. Once we had the data available and structured, we needed help extracting segmentations of the brain tumours, this is where CMIH and a lot of luck came in.
Liam Burrows, an expert in ML for segmentation, had some time available before starting a postdoc at Bath and was keen to help too. Having been affiliated to CMIH for nearly 5 years, I understood that this project fit in the scope of the Partnership Fund, to pump prime our project and allow us to build to further follow-on funding.
What is the aim of your project?
We had two simple aims. Firstly, to curate one of the largest datasets in the world for TYA brain tumour patients, consisting of MRI images pre- and post-operation. Secondly, to apply a range of existing adult brain tumour segmentation algorithms to the images and determine whether they performed well, or quantify where/how they fail.
How did the Partnership Fund help to achieve those aims?
The Partnership Fund provided the funds we needed to bring Liam onboard the team and to lead on the curation and segmentation of the dataset.
How do the aims of the CMIH fit with/alongside your work?
We are all very keen to ensure the benefits of ML are realised equitably. It was unclear whether models developed on 70+ year old patients would generalise to 12-16 year old patients, indeed we suspected they may not. Quantifying how under-served the TYA population would be a valuable outcome, and identifying gaps in current approaches.
What’s next for your project?
Now that we have curated the segmentation dataset, we have managed to recruit two additional team members to drive this project along. We will write up a manuscript describing the results of our analysis and build predictive models for outcome for the TYA patient group.