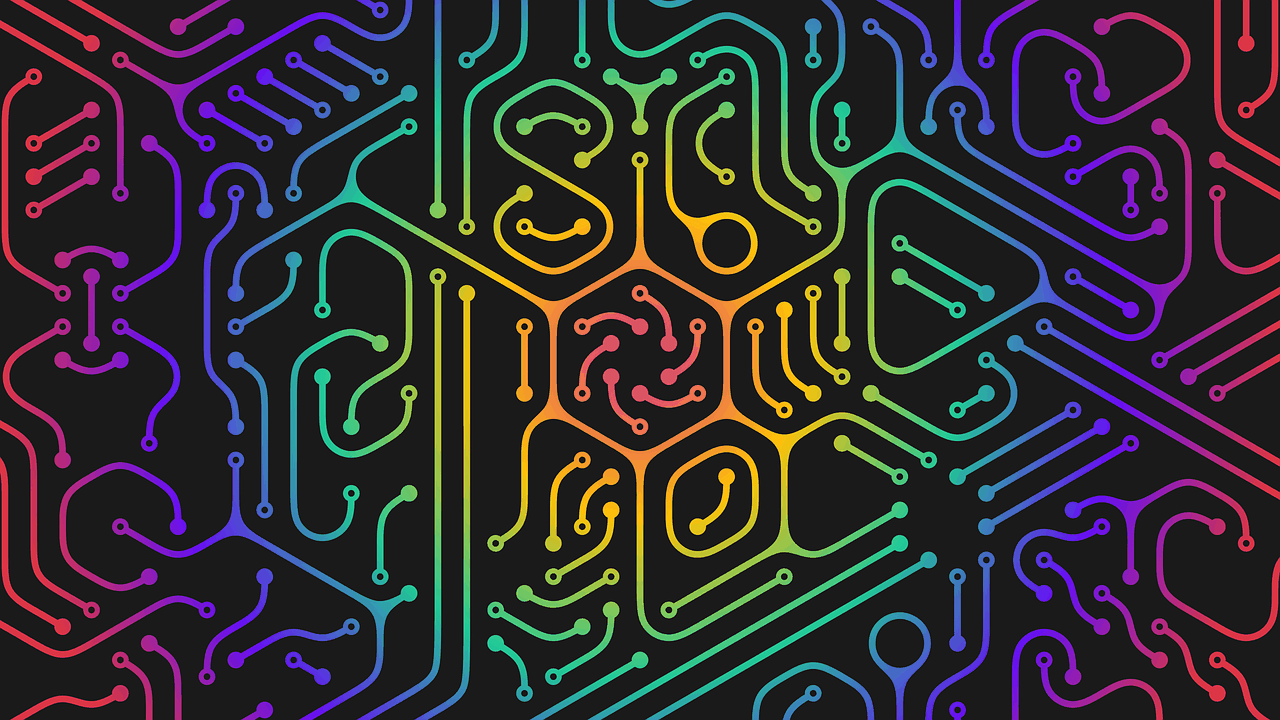
Submitted by Administrator on Thu, 01/06/2023 - 19:06
Machine learning is in a reproducibility crisis. Many codebases simply do not run when tested outside of the development environment and, even when they do run, many algorithms do not generalise outside of the dataset on which they are trained. In this paper, researchers from the CMIH Hub and AIX-COVNET teams argue that despite the democratization of powerful tools for data science and machine learning over the last decade, developing the code for a trustworthy and effective data science system (DSS) is getting harder. Perverse incentives and a lack of widespread software engineering (SE) skills are among many root causes we identify that naturally give rise to the current systemic crisis in reproducibility of DSSs. We analyze why SE and building large complex systems is, in general, hard. Based on these insights, we identify how SE addresses those difficulties and how we can apply and generalize SE methods to construct DSSs that are fit for purpose. We advocate two key development philosophies, namely that one should incrementally grow -- not biphasically plan and build -- DSSs, and one should always employ two types of feedback loops during development: one which tests the code's correctness and another that evaluates the code's efficacy.
Read the paper in full at:
Sören Dittmer, Michael Roberts, Julian Gilbey, Ander Biguri, AIX-COVNET Collaboration, Jacobus Preller, James H. F. Rudd, John A. D. Aston & Carola-Bibiane Schönlieb, (2023), Navigating the development challenges in creating complex data systems, Nature Machine Intelligence, https://www.nature.com/articles/s42256-023-00665-x