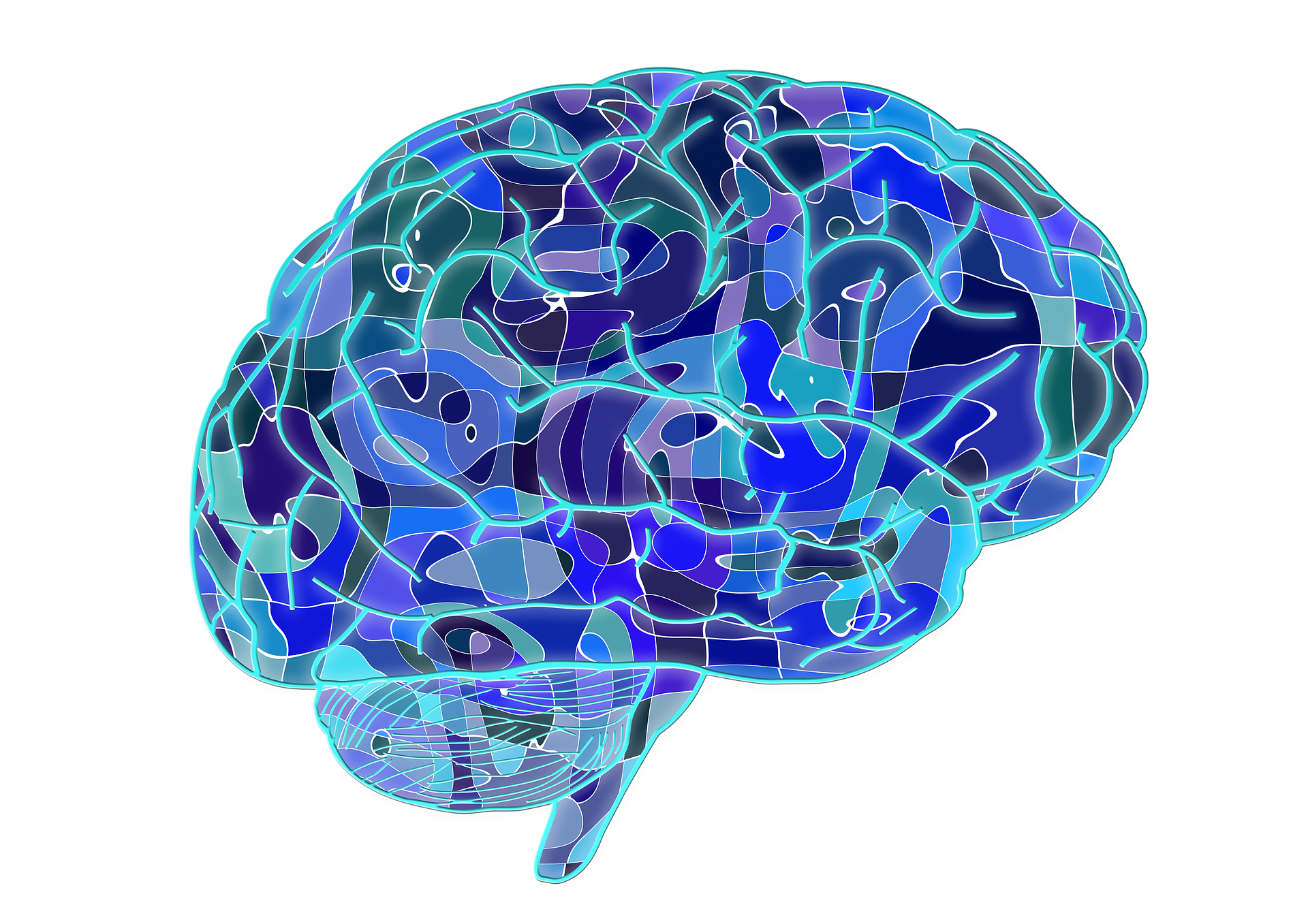
Submitted by Administrator on Tue, 23/05/2023 - 19:07
Glioma is the most common malignant brain tumour in adults. These tumours often vary between people resulting in very different outcomes.One of the most significant markers for the diagnosis and prognonsis of glioma is a mutation of the isocitrate dehydogenase (IDH) gene. Studies have shown that MRI can predict the genotype of glioma using machine learning or deep learning models however these can have their limitations.
Researchers from the CMIH Hub and colleagues have developed a novel learning framework tailored to characterise brain tumour and predict genotype in glioma. The research team proposes a multi-modal learning framework using three separate encoders to extract features of focal tumour image, tumour geometrics and global brain networks. To mitigate the limited availability of diffusion MRI, they developed a self-supervised approach to generate brain networks from anatomical multi-sequence MRI. Moreover, to extract tumor-related features from the brain network, they designed a hierarchical attention module for the brain network encoder. Further, they designed a bi-level multi-modal contrastive loss to align the multi-modal features and tackle the domain gap at the focal tumour and global brain. Finally, the research team proposes a weighted population graph to integrate the multi-modal features for genotype prediction. Experimental results on the testing set show that the proposed model outperforms the baseline deep learning models. The ablation experiments validate the performance of different components of the framework. The visualized interpretation corresponds to clinical knowledge with further validation. In conclusion, the proposed learning framework provides a novel approach for predicting the genotype of glioma.
You can read the paper in full at:
Yiran Wei, Xi Chen, Lei Zhu, Lipei Zhang, Carola-Bibiane Schönlieb, Stephen Price, Chao Li: Multi-modal learning for predicting the genotype of glioma, 2023, IEEE Transactions on Medical Imaging, doi: 10.1109/TMI.2023.3244038.