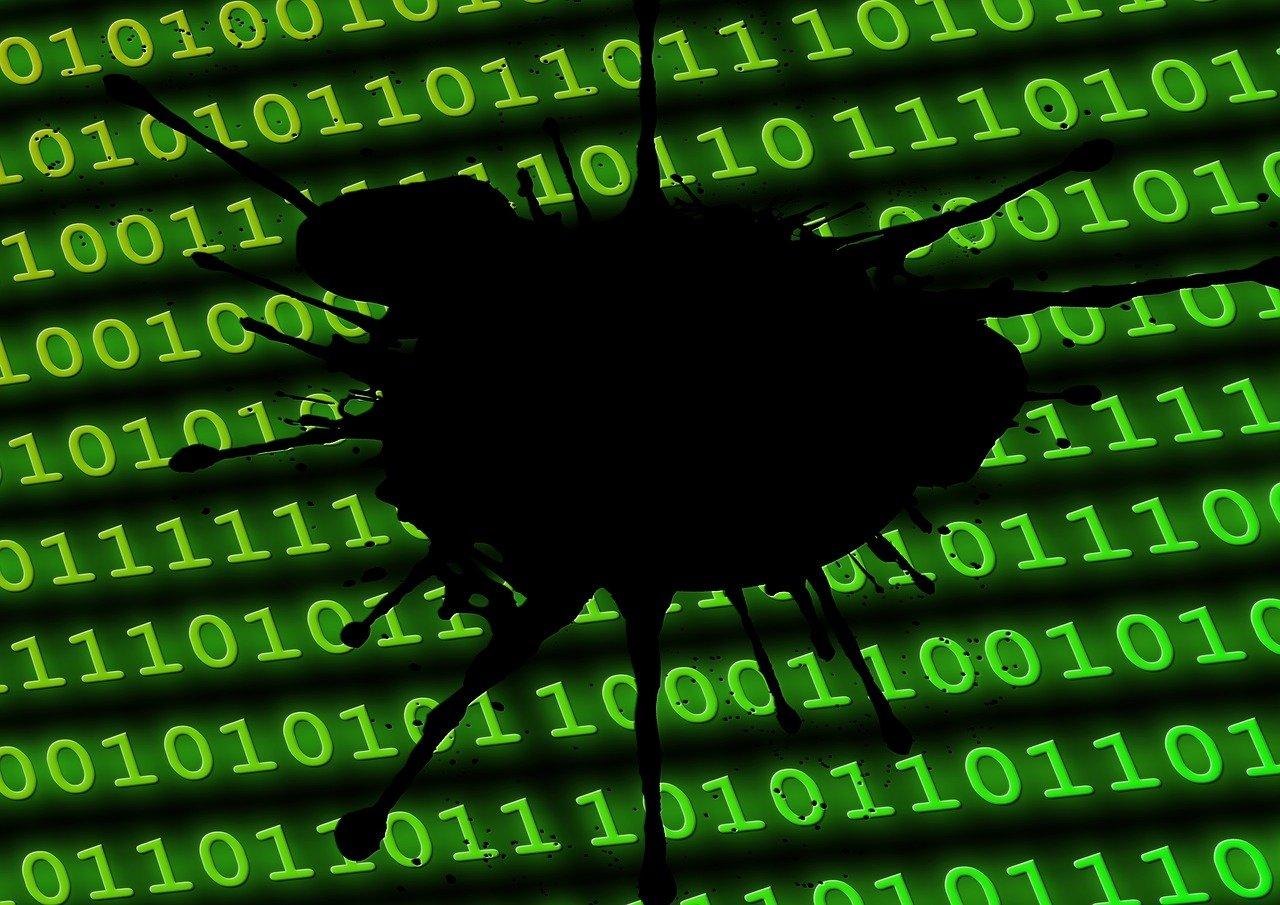
Submitted by Administrator on Fri, 06/10/2023 - 19:00
Classifying samples in incomplete datasets is a common aim for machine learning practitioners, but is non-trivial. Missing data is found in most real-world datasets and these missing values are typically imputed using established methods, followed by classification of the now complete samples. The focus of the machine learning researcher is to optimise the classifier’s performance.
Using three simulated and three real-word clinical datasets with different feature types and missingness patterns, researchers from AIX-Covnet and the CMIH Hub carried out the following research:
- evaluated how the downstream classifier performance depends on the choice of classifier and imputation methods
- employed ANOVA to quantitatively evaluate how the choice of missingness rate, imputation method, and classifier method influences the performance
- compared commonly used methods for assessing imputation quality and introduce a class of discrepancy scores based on the sliced Wasserstein distance
- assessed the stability of the imputations and the interpretability of model built on the imputed data
It was found that the performance of the classifier is most affected by the percentage of missingness in the test data, with a considerable performance decline observed as the test missingness rate increases. The team also showed that the commonly used measures for assessing imputation quality tend to lead to imputed data which poorly matches the underlying data distribution, whereas their new class of discrepancy scores performs much better on this measure. Furthermore, the team showed that the interpretability of classifier models trained using poorly imputed data is compromised.
Read more about this work in Nature Communications Medicine.
Shadbahr, T., Roberts, M., Stanczuk, J. et al. The impact of imputation quality on machine learning classifiers for datasets with missing values. Commun Med 3, 139 (2023). https://doi.org/10.1038/s43856-023-00356-z